Cambridge Data Week 2020 was an event run by the Office of Scholarly Communication at Cambridge University Libraries from 23–27 November 2020. In a series of talks, panel discussions and interactive Q&A sessions, researchers, funders, publishers and other stakeholders explored and debated different approaches to research data management. This blog is part of a series summarising each event.
The rest of the blogs comprising this series are as follows:
Cambridge Data Week day 1 blog
Cambridge Data Week day 3 blog
Cambridge Data Week day 4 blog
Cambridge Data Week day 5 blog
Introduction
Reuse of data is the final element of the FAIR principles and has long been argued as a central benefit of data sharing, allowing others access to a wealth of research and making research funding more efficient by removing the need to duplicate work. Yet we are still in the process of evaluating success in this area. This webinar brought together speakers to discuss what we know about the current state of play around data reuse, what researchers can do to increase the reuse potential of their data, and possible future developments in data reuse.
Our speakers – Louise Corti (UK Data Archive) and Tiberius Ignat (Scientific Knowledge Services) – looked at data reuse from two different perspectives. Louise focused on the reuse of UK Data Service collections, sharing some examples of their most widely used data sets, discussing what makes them popular and sharing some principles that can be used both to make data more reusable and to promote it for reuse. Tiberius discussed the prevalence of data reuse by machines and the possibility of granting machines data reuse rights.
Louise’s presentation gave an overview of the portfolio of data sets hosted by the UK Data Service, looked at their top 20 most downloaded datasets and discussed the underlying principles that have led to them being widely reused. As well as demonstrating some commonalities between these datasets, Louise also outlined the principles used by the UK Data Service to promote their collections for reuse.
Tiberius’ presentation looked at data reuse from a different perspective, serving as a call to action to share research data responsibly and protect it against the reuse of machines designed to persuade humans. One of Tiberius’ main arguments was that no research data from public projects should be made available to feed and develop persuasive algorithms.
The presentations motivated an interesting discussion covering a broad range of topics. These included the reuse of qualitative data, how we can implement ethical safeguards data reuse, the idea of data ethics as a continuum, whether we can accept positive cases of algorithmic persuasion such as to promote equality and diversity, and the possibility of creating specific licences prohibiting data reuse by persuasive algorithms. See below for a video and transcript of the session.
Audience composition
We had 341 registrations with just over 65% originating from the Higher Education sector. Researchers and PhD students accounted for nearly 37% of the registrations whilst research support staff accounted for an additional 33%. We also had registrations from at least 30 countries outside of the UK including significant attendance from Denmark, Holland, Germany and Canada. We were thrilled to see that on the actual day 187 people attended the webinar.
We held five online webinars during Cambridge Data Week and were pleased to see that nearly 25% of the participants attended more than one webinar. A total of 1364 people registered and more than 700 attended all together, with the rest possibly watching the recordings at a later date. Most of all we were pleased to welcome participants from all over the world and see how important research data management topics are globally.
Where data was available, we identified the following countries apart from the UK: Australia, Austria, Bangladesh, Brazil, Canada, Colombia, Croatia, Czech Republic, Denmark, France, Germany, Greece, Holland, Hungary, Iran, Luxembourg, Moldova, Norway, Poland, Romania, Singapore, Spain, Sweden, Switzerland, Turkey, Ukraine and the USA.
Recording , transcript and presentations
The video recording of the webinar can be found below and the recording, transcript and presentations are present in Apollo, the University of Cambridge repository.
Bonus material
After the session ended, we continued the discussion with Louise and Tiberius looking in particular at one question posed by an audience member:
AI can always be used either for good or bad. Instead of locking-in, how can we enhance technology through data and regulation?
Tiberius Ignat I think at this point we need regulation. I’m not a big fan of using regulations, to be honest. I think it’s much better to motivate people but, in this case, it’s quite a bit of control that has been lost, so I think we should have a regulation on how research data can be reused by others. This is how the internet has been made profitable during the last decade — through non-human persuasion. All these companies that are giving so much away for free are making billions of dollars when you look at the stock market. We were not clear how they were making this profit until recently when we realised that they are doing it by changing our behaviour and I think the rest of society – including research organisations – are behind them, so we need some regulation.
A good example is with GDPR. It has been introduced to protect our data, our digital footprint. On ResearchGate or Eurosport, or any other website, we used to be asked to agree to cookies or not. Recently, a new option called “Legitimate interest” has been slipped in and our digital data is again collected – less noticeably – by invoking questionable legitimate rights. The organisations whose model is based on persuading need cookie data, so they have moved the discussion away from remaining GDPR compliant to defending their legitimate interests. They are fighting to take data away from us. We can tackle this with regulation faster but in the long term we need to educate people to be more aware. We do have licenses such as Creative Commons but I’m not sure we have the right ones to protect us.
Louise Corti There are a variety of licenses, but they are abused and it’s very hard to track along the way what has gone wrong. I quite like the UK Government’s approach with some of their statistical data that has to go through a legal gateway. Some data can be made available for research, but it has to be done for the public good. We also have the Ethics Self-Assessment Tool, which is a grid you go through provided by the Statistics Authority and it asks you to think along lots of different dimensions of ethics. This helps researchers get a better sense of what they are trying to do, but whether the people we are talking about would care about it is a very different matter. Having been in research ethics for a very long time, that is by far the best tool I’ve seen and I recommend everyone uses it. The UK Data Archive uses it to evaluate some of the projects we deal with because you find often university ethics approvals are not good enough for the Statistics Authority because often they don’t understand quantitative secondary analysis, so the ethics scrutiny is not good enough. Self-Assessment is a much more nuanced thinking about the different dimensions of ethics and it helps researchers to be a bit more reflective about what’s good and what’s not.
Conclusion
Overall, the session provided a compelling blend of both the practical and conceptual elements of data reuse, each raising questions which could have easily been entire sessions in themselves. Louise’s presentation gave an excellent overview of the UK Data Service’s approach to making their datasets more reusable and promoting them to maximise their chances of being reused. Tiberius’ session raised some interesting questions surrounding data reuse and the ethics of using algorithms to persuade humans, as well as looking at some practical options for protecting research data from reuse for nefarious ends. At the end of the session, the audience were asked to participate in a poll on “What future developments are needed to increase the prevalence of data reuse?”.
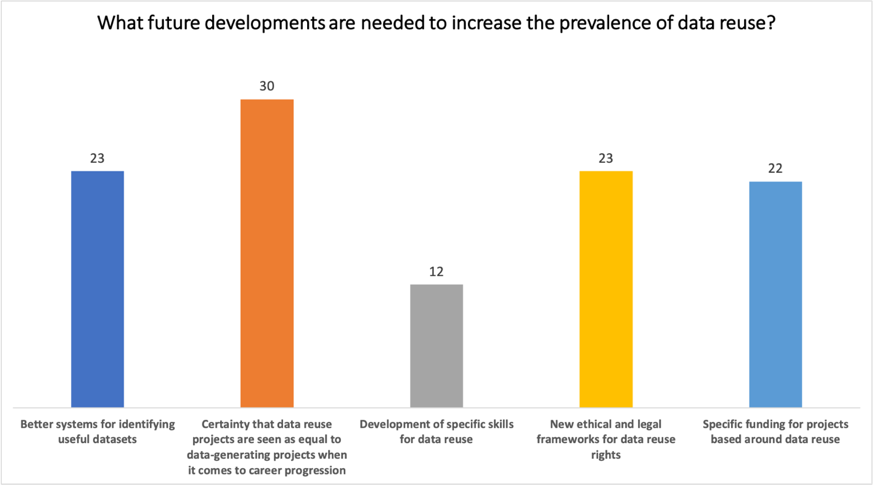
The results were unsurprising to either speaker, with each touching on the idea that a change in research culture is necessary to ensure data reuse projects are seen as equal to data-generating projects. The need for cultural change is a theme that ran throughout each of the sessions in Data Week and is perhaps one of the current major challenges in scholarly communication.
Resources
Robots appear more persuasive when pretending to be human
Behavioural evidence for a transparency–efficiency tradeoff in human–machine cooperation
The next-generation bots interfering with the US election
IBM’s AI Machine Makes A Convincing Case That It’s Mastering The Human Art Of Persuasion
Published on 25 January 2021
Written by Dominic Dixon
