In our third instalment of the Data Diversity Podcast, we are joined by Dr Nick H. Wise, Research Associate in Architectural Fluid Mechanics at the Department of Engineering, University of Cambridge. As is the theme of the podcast, we spoke to Nick about his experience as a researcher, but this is a special edition of the podcast. Besides being a scientist and an engineer, Nick has made his name as a scientific sleuth who, based on an article on the blog Retraction Watch which was written in 2022, is responsible for more than 850 retractions, leading Times Higher Education to dub him as a research fraudbuster. Since then, through his X account @Nickwizzo, he has continued his investigations, tracking cases of fraud and in some cases, naming and shaming the charlatans. Nick was kind to share with us many great insights over a 90-minute conversation, and as such we have decided to release a four part-series dedicated to the topic of research integrity.
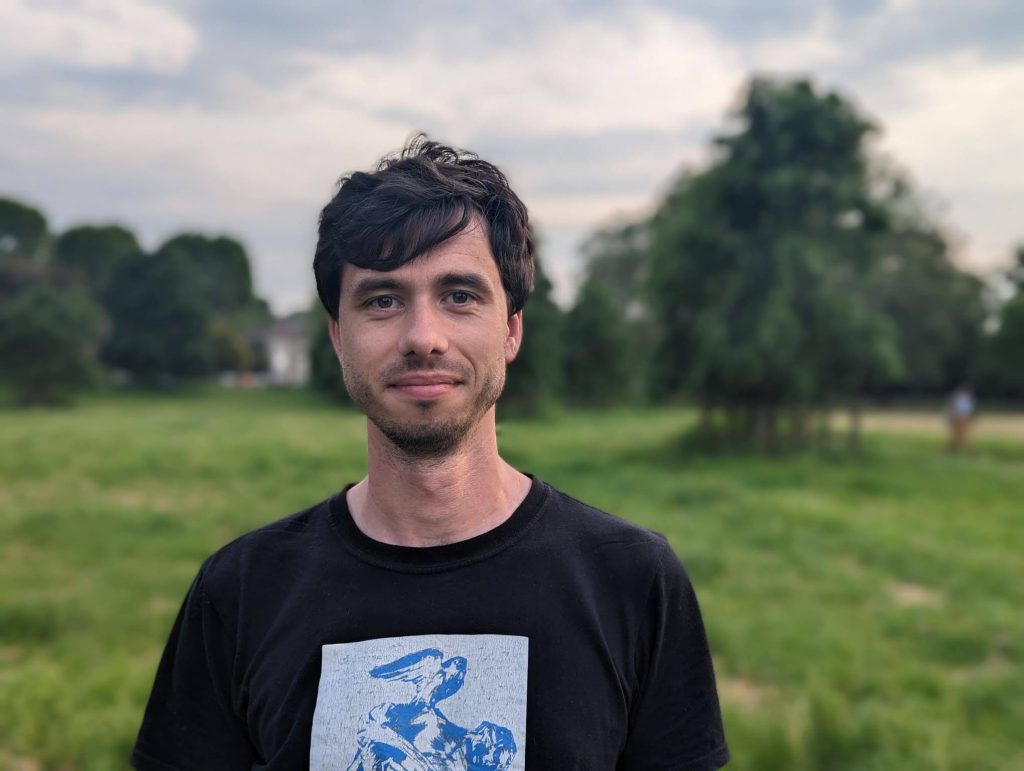
In this four-part series, we will learn from Nick about some of the shady activities that taint the scientific publishing industry today. In part one, we learn how Nick was introduced into the world of publication fraud and how that led him to investigate the industry behind it. Below are some excerpts from the conversation, which can be listened to in full here.
I have found evidence of a papermill bribing some editors and there have been many, at least tens, if not hundreds, of editors that have been let go or told to stop being editors by journals in the last year because they have been found to be compromised. This could be because of bribery or some other way of being compromised. This is what I try to uncover. – Dr Nick H. Wise
Tortured Phrases and PubPeer: Nick’s beginnings as a Scientific Sleuth
My background is in fluid dynamics where I mostly think about fluid dynamics within buildings. For instance, I think about the air flows generated by different heating systems and things like pollutant transport such as smells or COVID which can travel with the air and interact with other each other. That was my PhD and the post-doc in the Engineering department.
About three years ago whilst trying to avoid writing my thesis, I saw a tweet from the great Elizabeth Bik, who is possibly the most famous research fraud investigator. She mostly looks at biomedical images and her great skill is she would be able to look through a paper and see photos of Western blots of microscopy slides and see if parts of an image are identical to other parts, or if the image overlaps with images from different papers. She has an incredible memory and ability to spot these images. She’s been doing this for over 10 years and has caused many retractions. I was aware of her work but there was no way for me to assist with that because it is not my area of research. I don’t have an appreciation of what these images should look like.
But about three years ago she shared a preprint written by three computer scientists on her Twitter account about a phenomenon they called ‘tortured phrases’. In doing their research and reading the literature, these computer scientists noticed that there were papers with very weird language in them. What they surmised was that to overcome plagiarism checks by software like Turnitin, people would run text through paraphrasing software. These software were very crude in that they would go word by word. For instance, it would look at a word and replace it with the first synonym it found in a thesaurus. It would do this word for word, which makes the text barely readable. However, it is novel and so it will not flag any plagiarism checking software. Eventually, if you as a publisher have outsourced the plagiarism checks to some software, and neither your editor or peer reviewer reads the text to check if it makes sense, then this will get through peer review process without any problem and the paper would get published.
For an example of tortured phrases: sometimes there’s not only one way to say something. Particularly if English is not someone’s first language, you don’t want to be too harsh on anyone who’s just chosen a word which just isn’t what a native speaker would pick. But there are some phrases where there’s only one right way to say it. For instance, artificial intelligence is the phrase for the phenomenon you want to talk about, and if instead you use “man-made consciousness”, that’s not the phrase you need to use, particularly if the original text said artificial intelligence brackets AI, and your text says “man-made consciousness” brackets AI. It’s going to be very clear what has happened.
The three computer scientists highlighted this phenomenon of ‘tortured phrases’, but entirely from within the computer science field. I wondered if a similar phenomenon was happening in my own field in fluid dynamics. Samples of these paraphrasing software are freely available online as little widgets so I took some standard phrases from fluid dynamics, which were the kind that would not make sense if you swapped the words around and generated a few of these tortured phrases, I googled them and up popped hundreds of papers featuring these phrases. That was the beginning for me.
I started reporting papers with these phrases on a website called PubPeer, which is a website for post-publication peer review. I commented on these papers and started being in conversation with the computer scientists who wrote the paper on ‘tortured phrases’ because they built a tool to scrape the literature and automatically tabulate these papers featuring these phrases. They basically had a dictionary of phrases which they knew would be spat out by the software because some of this paraphrasing software are so crude, such that if you put in “artificial intelligence”, you are always going to get out “man-made consciousness” or a handful of variants. It didn’t come up with a lot of different things. If you could just search for “man-made consciousness” and it brings up many papers, you knew what has been going on. I contributed a lot of new ‘fingerprints’, which is what they call their dictionary that they would search the literature for. That is my origin story.
On Paper Mills and the Sale of Authorships
There is also the issue of meta-science, which has nothing to do with the text of the paper or with the data itself, but more to do with how someone may add a load of references through the paper which are not relevant, or they are all references to one person or a colleague. In that way you would be gaming the system to boost profiles, careers, and things like H-index. Because having more publications and more citations is so desirable, there is a market for this. It is easy to find online advertisements for authorship of scientific papers ranging from $100 to over $1000, depending on the impact factor of the journal, and the position of authorship you want: first authorship, seventh authorship, or whether you want to be the corresponding author, these sorts of factors. Likewise, you can buy citations.
There are also organizations known as paper mills. For example, as an author I might have written the paper and want, or need, to make some money and so I go to this broker and say: I want to sell authorships, I’ll be author number six, but I can sell the first five authorships. Can you put me in touch with someone selling authorships? At the same time, there are people who go to them saying I want to buy an authorship, and they put two and two together acting as a middleman. Also, some of these paper mills do not want to wait for someone to come to them with a paper – they will write papers to order. They have an in-house team of scientific writers who produce papers. This does not necessarily mean that the paper is bad. Depending on where they want the paper to publish, the paper might have to be good if it has to get published. So, they will employ people with degrees, qualified people or PhD students who need to earn some money, and then they will sell the authorships and get the papers published. This is a big business.
There is a whole industry behind it, and something I have moved onto investigating quite a lot is where these papers are going. When I identify these papers, I try to find out where they are being published, how they’re being published, who is behind them, who is running these paper mills, who is collaborating with them. Something I found out which resulted in an article in Science was that paper mills want to guarantee acceptance as much as they can. If a paper is not accepted, it creates a lot of work for them and it means a longer time before their customers get what they paid for. For example, if a paper that they wrote and sold authorships for gets rejected, they’re going to have to resubmit it to another journal. So something paper mills will do is they will submit a paper to 10 journals at once and publish with whichever journal gave them the easiest time. But still, they want to try and guarantee acceptance and one way to do that is to simply bribe the editor. I have found evidence of a papermill bribing some editors and there have been many, at least tens, if not hundreds, of editors that have been let go or told to stop being editors by journals in the last year because they have been found to be compromised. This could be because of bribery or some other way of being compromised. This is what I try to uncover.
Although I’m not fighting this alone, it can feel like that. Publishers are doing things to some extent and they’re doing things that they can’t tell you about as well. And then there’s other people like me investigating this in their free time or as a side project. Not enough of us are doing it because it is a multi-million-dollar industry that is generating these papers. More papers are being published than ever before so it is a big fight.
Stay tuned as we release the rest of the conversation with Nick over the next month. In the next post, we get Nick’s take on the peer review process and fake research data, and I ask his opinion on where the fault lies in the publication of fraudulent research.